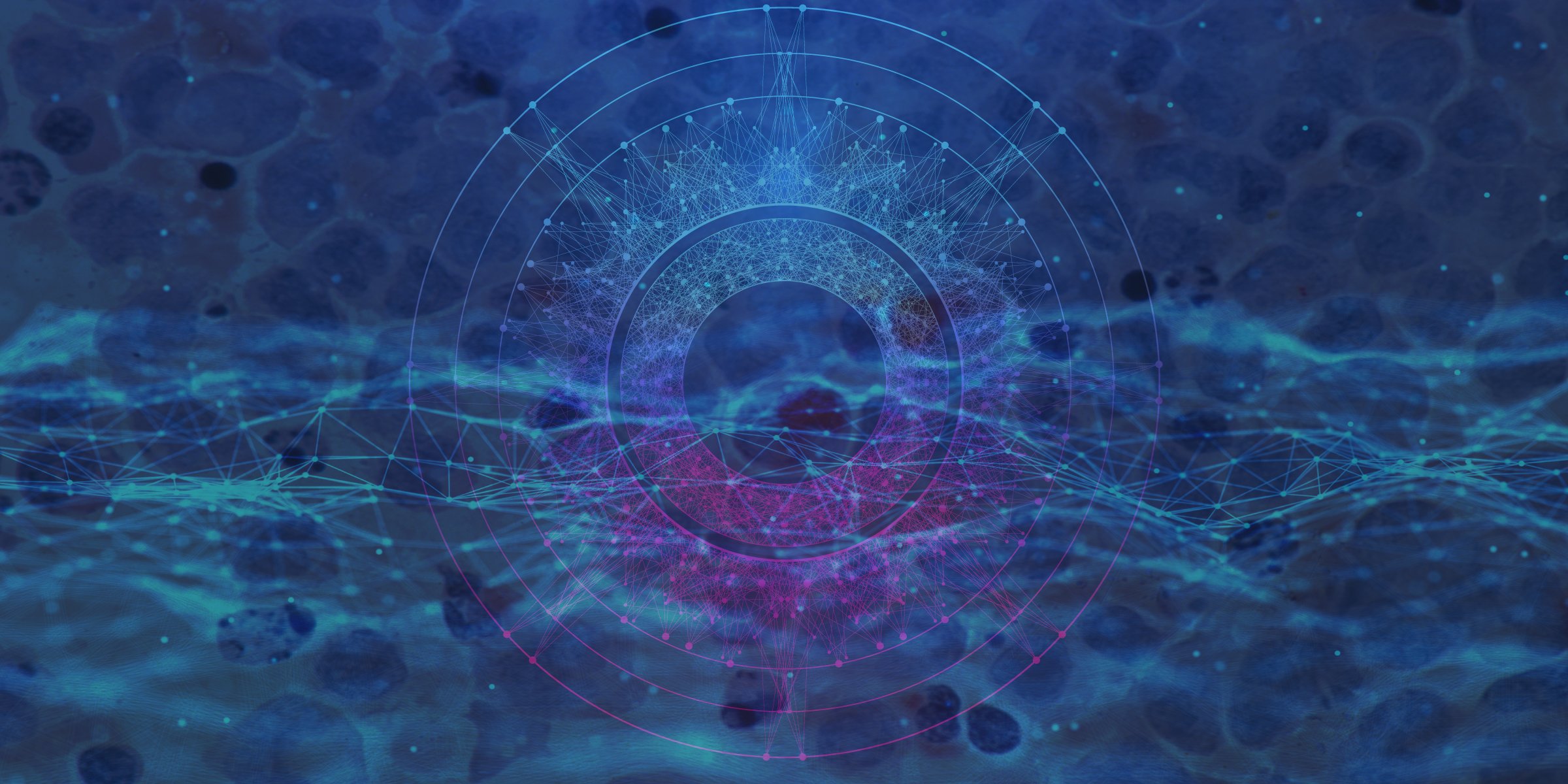
Our
Research
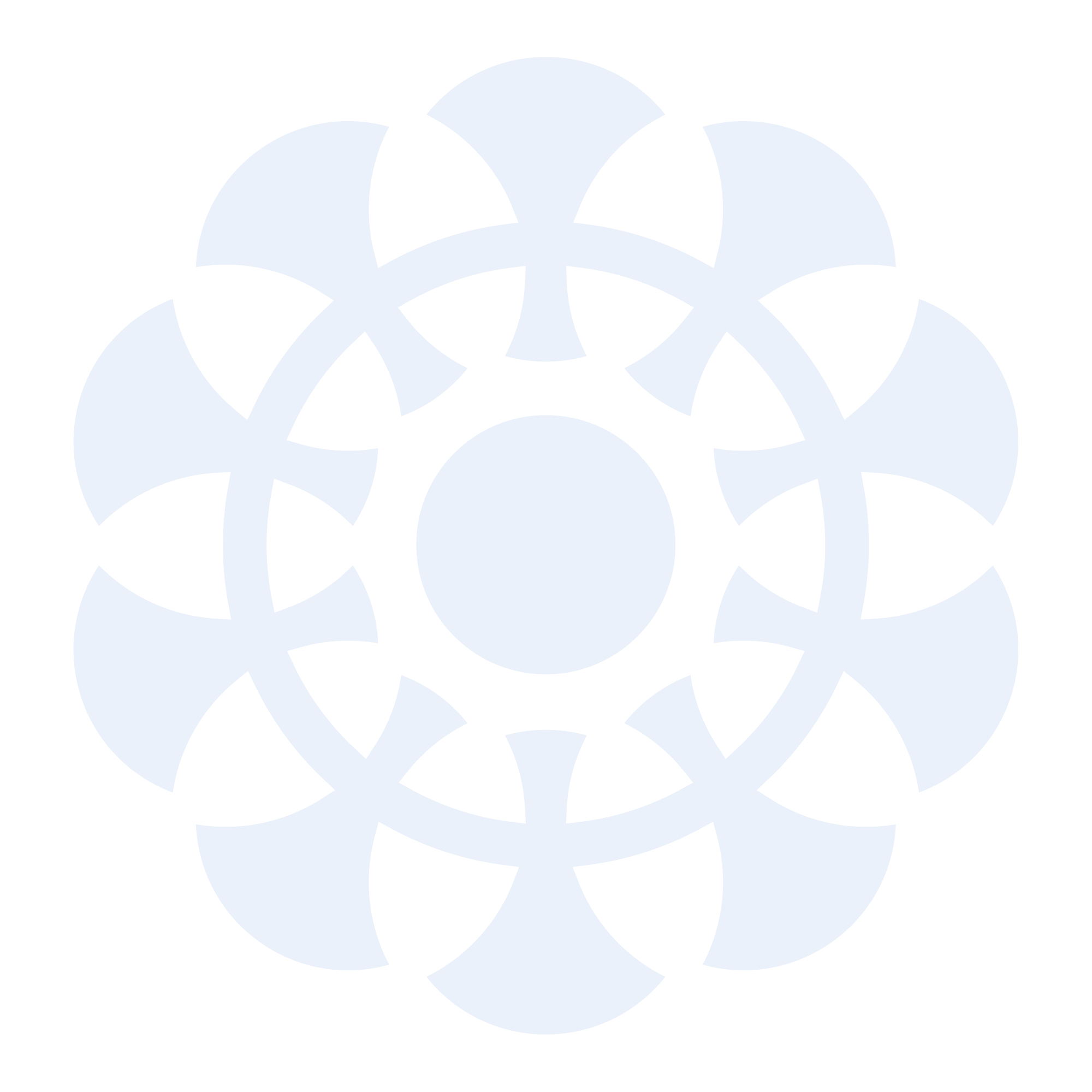
Research at the Rabadan Lab
Our main scientific interests lie in modeling and understanding the dynamics of biological systems through the lens of genomics. We are a very interdisciplinary team of mathematicians, physicists, engineers, biologists, and medical doctors with a common goal of solving pressing medical problems. We are currently focusing our work on:
Cancer. Genomic technologies provide an extraordinary opportunity to identify mutations that contribute to the development of tumors. We are mapping the evolution of cancers and uncovering the mechanisms of response or lack of response to multiple therapies. We work with clinicians and experimentalists all around the world.
Infectious diseases. Evolution is a dynamic process that shapes genomes. Our team at Columbia is developing algorithms to analyze genomic data, with a view to understanding the molecular biology, population genetics, phylogeny, and epidemiology of viruses. We are interested in the emergence of infectious diseases, pandemics and uncovering the mechanisms of adaptation of viruses to humans.
Electronic Health Records. Clinical databases constitute a rich and complex source of raw data. We are using the power of statistics and computers to tease out important clinical patterns in these diverse, important datasets. Combining molecular and clinical data illuminates some of the mechanisms underlying complex diseases.
In particular, we develop mathematical, statistical, and computational approaches, which cover the analysis of high throughput data right through to the altogether more abstract identification of global patterns in evolutionary processes. Learn more about the Rabadan Lab and the three main global questions that we are addressing.
Research Projects
Computing The Role Of Alternative Splicing In Cancer
Accumulating evidence indicates that recurrent spliceosomal mutations contribute to the initiation and progression of several cancers through diverse fundamental cellular processes.A number of computational tools are used to characterize splicing effects in cancer. These tools present limitations that can be overcome by running alternative splicing analysis with multiple tools and integrating the results.Extracting splicing events functionally relevant to cancer requires rigorous quality control to filter technical artifacts, cross-validate the events using independent datasets, and integrate alternative approaches including regulatory network characterization and cancer signaling pathway analyses. By taking advantage of the increasing amount of genomic data, deep learning-based methods have dramatically improved the state-of-the-art performance of alternative splicing analysis.